Avoid AI Disasters: 5 Critical Evaluations for a Responsible AI System Launch
- Aleksandar Jevtic
- 4 days ago
- 12 min read
Artificial Intelligence demonstrates great potential within healthcare and finance, together with additional vital sectors. The transformative power of artificial intelligence becomes visible through its ability to speed up drug development alongside customized medical treatments, financial prediction enhancement, and HR process optimization. Organizations of all sizes are currently racing to deploy predictive AI together with generative AI (GenAI) and sophisticated agentic AI systems while facing substantial risks that include biased outcomes, security vulnerabilities, regulatory penalties and public trust degradation.
As CEO and Chief AI Ethics Officer (CAIEO) of TrustVector, AI Ethics Authorized Lead Assessor, Trainer and Evaluator for the IEEE Standards Association CertifAIEd™ Program, and with two decades of experience leading health industry digital transformations, I have directly observed the negative effects of rushing innovation without proper planning. Launching an AI system represents both a technological achievement and a serious strategic decision which creates major ethical and operational implications.
Here are five critical evaluations you must conduct before launching your AI system responsibly:
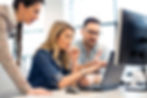
1. Strategic Alignment & Comprehensive Impact Assessment
Any AI project should start by clarifying its core purpose and examining potential outcomes.
Define purpose and scope. Identify the specific goals of your AI system together with its designated applications and expected organizational outcomes. [2] Your organization's entire strategy along with its core values should guide any AI initiative. Vague objectives don’t help. They don’t provide enough guidance to developers to create quality systems, and make it very difficult to measure true success or properly evaluate risk. You should aim for specificity when defining the objectives of your AI system.
For example, if your AI system’s goal is to enhance pediatric asthma care, then the specific objective may be: “Identify pediatric patients (ages 5-17) with moderate-to-severe asthma who are at high risk (defined as >70% probability) of experiencing an exacerbation requiring an emergency department visit or hospitalization within the next 14 days, enabling proactive intervention by care teams. Predictive AI models will be validated using routinely collected electronic health record (EHR) data (including demographics, diagnoses, medications, lab results) and wearable sensor data (monitoring respiratory rate and activity levels).”
If an AI system is designed for loan application processing, the objective could be: “To automate the initial loan application review, reducing processing time by 50%, while ensuring compliance with the Equal Credit Opportunity Act and avoiding bias against applicants from specific demographic groups.”
For AI-driven talent acquisition, an objective might be: “To streamline the recruitment process by automatically screening resumes, reducing time-to-hire by 30%, while ensuring diversity and inclusion by mitigating potential biases in the selection algorithm.”
Clarity is key.
Conduct AI impact assessments. This is crucial, especially for systems deemed high-risk under well established AI risk management frameworks or regulations such as the EU AI Act.[20] Systematically identify who could be harmed by the AI, including vulnerable populations often overlooked.[2] For instance, when developing AI for pediatric mental health, we need to recognize both the special exposures to and impacts on children and their families.[14] The assessment should include both direct and indirect effects the system could produce. What backup procedures are in place for cases when the AI system performs incorrectly? Active and early participation of clinicians, patients, ethicists and legal experts throughout the process is essential to properly anticipate needs and challenges you may encounter as you work towards building trust in your AI systems.[14], [4] AI applications in sectors like finance for credit scoring or fraud detection present significant risks of bias and financial harm, while in HR, automated hiring tools can perpetuate existing inequalities if not carefully assessed.
Use established frameworks. There are many frameworks to choose from that will get you on the right track. You can use the NIST AI Risk Management Framework (AI RMF)[18] or IEEE Standards Association CertifAIEd™ Program[17] or the OECD AI Principles[16], to mention a few, to develop your assessment structure. These frameworks enable organizations to determine AI risks through standardized approaches for assessment and control which both meets regulatory standards and follows industry best practices. This is by no means an exhaustive list of available frameworks, and choosing the right framework for your organization is a step that will require some effort and strategic thinking. The key is to recognize that you don’t have to start from scratch.
The proactive evaluation of impact combined with strategic alignment leads to the development of valuable AI solutions such as those genuinely advancing precision medicine[14] instead of future liabilities. The process protects organizations from investing resources into systems that could result in reputational damage or costly remediation expenses in the future.
2. Robust Data Governance & Quality Assurance
AI models acquire their fundamental characteristics from the training data they receive. Flawed data will always produce flawed, biased or unreliable results from AI systems.
Establish stringent data quality standards. Clearly document data accuracy, format, relevance, and completeness requirements prior to model training.[7] Implement data cleaning procedures and ensure that you have established a process for missing value handling and outlier management to prevent biased results.[8] AI requires high-quality representative data especially when working with complex unstructured data sets, such as those frequently encountered in healthcare.[14], [15] In finance, this means ensuring that data used for risk assessment models is accurate, complete, and free from biases that could lead to discriminatory lending practices. In HR, it involves safeguarding employee data used for performance evaluations and ensuring fairness in training data for recruitment AI.
Prioritize bias detection and mitigation. The training data requires thorough bias examination followed by implementation of mitigation strategies to prevent discriminatory results.[2] A thorough evaluation of data representativeness together with the implementation of mitigation techniques (e.g., resampling and algorithmic adjustments) becomes necessary during the development stage.[2] Regular fairness audits are essential. In predictive AI systems that perform loan applications and clinical risk scoring operations this requirement is critical to avoid amplifying social inequalities. The requirement of fairness in AI-based underwriting systems, for example, demands strict bias mitigation protocols.[14]
Ensure data privacy and security. The implementation of strong privacy and security measures should extend across the entire AI lifecycle through encryption, access control mechanisms, and privacy-enhancing techniques like differential privacy.[4] The organization must adhere to all applicable data protection standards (e.g., GDPR, HIPAA) and conduct regular data protection impact assessments.[4] Innovation with AI needs to be carefully managed in relation to data privacy concerns, particularly in healthcare settings.[14] Data security sharing protocols represent a critical requirement for joint AI research activities.[14] This is critical in finance, where data breaches could lead to severe financial losses and regulatory penalties, and in HR where protecting employee data is essential for maintaining trust and complying with labor laws.
Practice data minimization. The AI system should only process data that directly serves its designated function and avoid unnecessary information.[9] Organizations should perform periodic data reviews to eliminate records that no longer serve any purpose, and ensure that AI vendors that rely on your data do the same. This reduces the attack surface and minimizes privacy risks.[9]
In sensitive sectors like healthcare and finance, meticulous data governance isn't just a best practice; it's a fundamental requirement for building systems that are effective, fair, and worthy of trust.[14]
3. Rigorous Model Testing: Beyond Basic Accuracy
Using clean test data for model validation through standard accuracy metrics alone is both inadequate and hazardous. The evaluation process for real-world performance needs multiple extensive assessment methods.
Comprehensive performance evaluation. The evaluation of model performance requires multiple relevant metrics which include precision, recall, F1-score, ROC-AUC, along with accuracy.[8] Examine how well the model maintains consistent performance when dealing with different subgroups together with various deployment settings and data variations that might occur post-deployment.[4] A proper evaluation plan should include suitable test data that stays separate from training data and established performance benchmarks.[4] Consider the specific demands of the AI system you are creating, whether it's assisting surgeons or managing chronic conditions like pediatric asthma, and think carefully how you’ll measure the performance of your AI models.[14] When it comes to talent acquisition for example, performance evaluation could involve testing how the AI performs with applicants from diverse backgrounds or with varying work histories.
Stress testing and red teaming. The AI model needs active testing for discovering its potential weaknesses through stress testing for unusual conditions and adversarial testing (red teaming) for identifying vulnerabilities.[2] GenAI needs evaluation for prompt injection as well as data leakage, hallucination, and jailbreaking.[2] Agentic AI requires testing both autonomous decision-making boundaries and possible harmful unintended actions. The deployment of AI in critical infrastructure or healthcare requires red teaming as an essential component rather than an optional feature.[14],[2] In finance, red teaming could involve trying to manipulate fraud detection systems. In HR, it could involve testing if AI chatbots used for employee support provide biased or inappropriate advice.
Security fortification. This includes deploying protective measures at both system and application levels to defend the AI tool from malicious threats.[4]
Continuous monitoring. The evaluation process should continue after launch through planned continuous monitoring activities. The AI system requires constant performance monitoring together with drift detection and real-time bias assessment through deployed mechanisms.[2] AI systems which operate in dynamic environments, such as population health management, require this approach to preserve their reliability.[4]
A model which shows excellent performance in the lab may produce disastrous results when deployed in production environments. Real-world robustness and security depends on thorough testing that includes multiple approaches and simulations of actual operational scenarios and adversarial threats.
4. Ensuring Transparency, Explainability & Human Oversight
Clear understanding of AI system’s operational logic, i.e. how the system works, and human oversight are critical to building trust in the AI system’s recommendations / outputs. As the risk of human harm from the AI system increases, so does the need for transparency, explainability and human oversight. The practice of using “black box” AI systems becomes more and more unacceptable especially in the high-risk situations, such as healthcare or financial decisions.[14]
Maintain clear documentation. The requirement for complete documentation stands as an absolute necessity for both transparency and accountability purposes.[2] All documentation must include model source information and version details alongside training data specifics and intended use cases and limitations and performance evaluation results.[2] Model cards are becoming standard practice, and you should consider using them as part of your documentation activities.[10] Yes, this takes time, but it’s time well spent. I’ll go back to my software development roots and my education where code without proper documentation was essentially useless to anyone but the original developer. These are good practices that should not be forgotten and are now maybe even more important than before.
Provide transparency disclosures. Be clear when individuals are interacting with an AI system or when AI generates content. Users should receive notifications about their personal data usage for training purposes along with appropriate opt-out options when necessary.[2] The practice of providing clarity about AI systems contributes not only to increased trust in AI systems recommendations, but typically also fulfills legal requirements.[2] Achieving better understanding of AI use by everyone including patients, financial clients, and job applicants remains essential.[14]
Strive for explainability. The implementation of explainability methods like SHAP or LIME should occur whenever it is feasible and appropriate for decisions that carry substantial impact such as medical diagnosis and credit scoring.[2] Stakeholders need to understand why an AI reached a particular conclusion.[11] Efforts to "unveil the black box" are crucial for fostering trustworthy AI integration in healthcare decisions.[14] In financial services, providing explanations for loan denials is essential for compliance and customer trust. In HR, explaining why a candidate was not selected can help improve hiring practices and reduce legal risks.
Ensure adequate human oversight. Human-in-the-Loop must be implemented for high-risk applications because automated decisions need human oversight. Create mechanisms for human review and validation of AI outputs, and clear pathways to challenge the outcomes.[2] Dedicate time to properly define human intervention roles as these roles are integral to having safe and accountable AI systems. The EU AI Act sets forth human oversight as a clear requirement for high-risk systems, and your organization should maintain the same standard regardless of your geographic location.
Transparency isn't just about revealing algorithms; it's about fostering understanding, enabling scrutiny, and ensuring that humans retain ultimate control and accountability, particularly when the stakes are high.
5. Establishing a Robust Compliance & AI Governance Framework
Navigating the complex and fast-evolving AI regulatory and AI standards environment requires a structured governance approach.
Understand the regulatory environment. Knowing the AI regulations that apply to your organization has become an increasingly complex task. However, staying informed about applicable AI regulations, which vary by jurisdiction and risk level (e.g., EU AI Act [3], current and future US state and federal rules[13], industry-specific mandates), is a must. “Our legal team will take care of that” is not a good strategy. Partner with your legal team to achieve success, but don’t expect that they will lead the charge. Frameworks like the NIST AI RMF [5] or IEEE Standards Association CertifAIEd™ program, and standards like ISO/IEC 42001 [11], provide valuable guidance for structuring compliance efforts.[6] Identifying applicable regulations early is crucial, as the EU AI Act is proving to be a game changer for responsible AI development.[14], [4]
Adding to this complexity, organizations must also continue to comply with numerous well-established regulations unrelated to AI, such as Dodd-Frank and anti-money laundering laws for financial institutions, or labor laws and data privacy regulations for HR departments.
Implement internal AI governance. Organizations should create policies, ethical guidelines and compliance procedures to govern AI development and deployment.1 To guarantee both oversight and accountability, organizations should create well defined AI governance roles and responsibilities that could include positions like Chief AI Officer or an AI ethics committee.[12] All AI systems operating within the organization should be registered within a central inventory system.[2] The responsible management of powerful AI tools depends heavily on this internal organizational structure particularly when operating in highly regulated or high-risk industries like healthcare and finance.[14]
Manage third-party AI risk. Third-Party AI risk management requires thorough examination of all external AI models and tools.[2] Before using external models, APIs, or tools from organizations such as OpenAI, Google, Anthropic, or specialized vendors like those providing AI for surgical assistance[14], you must perform a thorough assessment of these third parties.[2] Inspect the model documentation, security certifications, AI governance practices, data usage terms and compliance posture maintained by these third parties.[2] Ensure contracts clearly define responsibilities.[2] For best results, ensure that third-party AI systems you may be relying on have been independently checked against known responsible AI frameworks and, ideally, have been independently certified.
Develop an AI incident response plan. Prepare for AI system failures by creating incident response protocols to handle biased outputs, data breaches and regulatory inquiries.[2] Test this plan regularly.
The processes of compliance and governance serve as fundamental organizational tools that drive both safe and innovative development. A proactive compliance and AI governance framework enables organizations to manage risk and maintain legal and ethical alignment which builds trustworthy AI adoption throughout their operations.[14]

Conclusion: Moving Forward Responsibly
AI deployment extends beyond technical requirements, and requires both strategic planning and responsible execution. An evaluation of strategic alignment combined with data governance, model robustness, transparency, and compliance assessment serves as the essential foundation for maximizing AI benefits and controlling its potential risks before launching.[14]
The foundation for building trust with your customers, your employees, regulators, and society at large lies in proactive evaluation of AI systems guided by frameworks like the NIST AI RMF and regulations such as the EU AI Act. It’s how we move from hype to tangible, responsible value creation.[14]
It's crucial to remember that you don't have to implement all of these measures simultaneously, and you don't have to undertake this task alone. Formulating a well-structured plan to progressively build the necessary capabilities within your organization over time, while concurrently executing identified tactics, is a viable and effective approach.
Look for outside help from experts, or collaborate with partner organizations that specialize in AI ethics and AI risk management. Their expertise enables organizations to gain valuable insights and navigate complex regulatory landscapes while helping to develop robust governance frameworks and perform vulnerability assessments, certification of AI systems, and ongoing monitoring.
Additionally, foster a culture of continuous learning and improvement within your organization. Encourage employees to stay updated on the latest developments in AI ethics, participate in relevant training programs, and proactively identify potential risks.
The development of responsible AI requires continuous effort. The implementation of proactive iterative approaches enables you to control risks while building stakeholder trust and delivering long-term AI benefits that maintain ethical standards.
Works cited
NIST AI and ISO 42001, and EU AI Act - TrainingTraining.Training, https://www.trainingtraining.training/blog/nist-ai-iso-42001-and-eu-ai-act-explained
The Ultimate AI Compliance Checklist for 2025: What Every Business Must Do Now, https://neuraltrust.ai/blog/ai-compliance-checklist-2025
White Papers 2024 Understanding the EU AI Act - ISACA, https://www.isaca.org/resources/white-papers/2024/understanding-the-eu-ai-act
FUTURE-AI: international consensus guideline for trustworthy and deployable artificial intelligence in healthcare | The BMJ, https://www.bmj.com/content/388/bmj-2024-081554
AI Risk Management Framework | NIST, https://www.nist.gov/itl/ai-risk-management-framework
Navigating AI Compliance: An Integrated Approach to the NIST AI RMF & EU AI Act - Securiti, https://securiti.ai/whitepapers/an-approach-to-nist-ai-rmf-and-eu-ai-act/
AI governance checklist (updated 2025) | Streamline oversight with a comprehensive AI governance checklist | AI cybersecurity & governance checklist insights | LLM best practices | Lumenalta, https://lumenalta.com/insights/ai-governance-checklist-updated-2025
Best Practices for AI Model Validation in Machine Learning - Galileo AI, https://www.galileo.ai/blog/best-practices-for-ai-model-validation-in-machine-learning
Responsible AI Checklist - - TrustArc, https://trustarc.com/wp-content/uploads/2024/05/Responsible-AI-Checklist-.pdf
Responsible AI Progress Report - Google AI, https://ai.google/static/documents/ai-responsibility-update-published-february-2025.pdf
Navigating the Future of AI Governance: A Guide to NIST AI RMF, ISO/IEC 42001, and the EU AI Act - ZenGRC, https://www.zengrc.com/blog/navigating-the-future-of-ai-governance-a-guide-to-nist-ai-rmf-iso-iec-42001-and-the-eu-ai-act/
AI Principles and Best Practices - ModelOp, https://www.modelop.com/ai-governance/ai-principles-and-best-practices
AI Regulations & Standards - ModelOp, https://www.modelop.com/ai-governance/ai-regulations-standards
Resources | TrustVector, https://www.trustvector.ai/blog
Preparing Healthcare Data for AI Models - Wolters Kluwer, https://www.wolterskluwer.com/en/expert-insights/preparing-healthcare-data-for-ai-models
The OECD AI Policy Observatory, https://oecd.ai/en/
IEEE SA CertifAIEd™ Program, https://standards.ieee.org/products-programs/icap/ieee-certifaied/
NIST AI Risk Management Framework, https://www.nist.gov/itl/ai-risk-management-framework
Mansoor, M.; Hamide, A.; Tran, T. Conversational AI in Pediatric Mental Health: A Narrative Review. Children 2025, 12, 359. https://doi.org/10.3390/children12030359
EU AI Act, https://eur-lex.europa.eu/legal-content/EN/TXT/?uri=CELEX%3A32024R1689